How can artificial intelligence be integrated into historically developed, complex inventory software in order to make processes more efficient? We got to the bottom of this question together with schrempp edv in one of our AI Ideation workshops. The result: an innovative approach to how AI can revolutionize the use of the ERP system SIVAS.ERP.
Artificial Intelligence meets legacy ERP
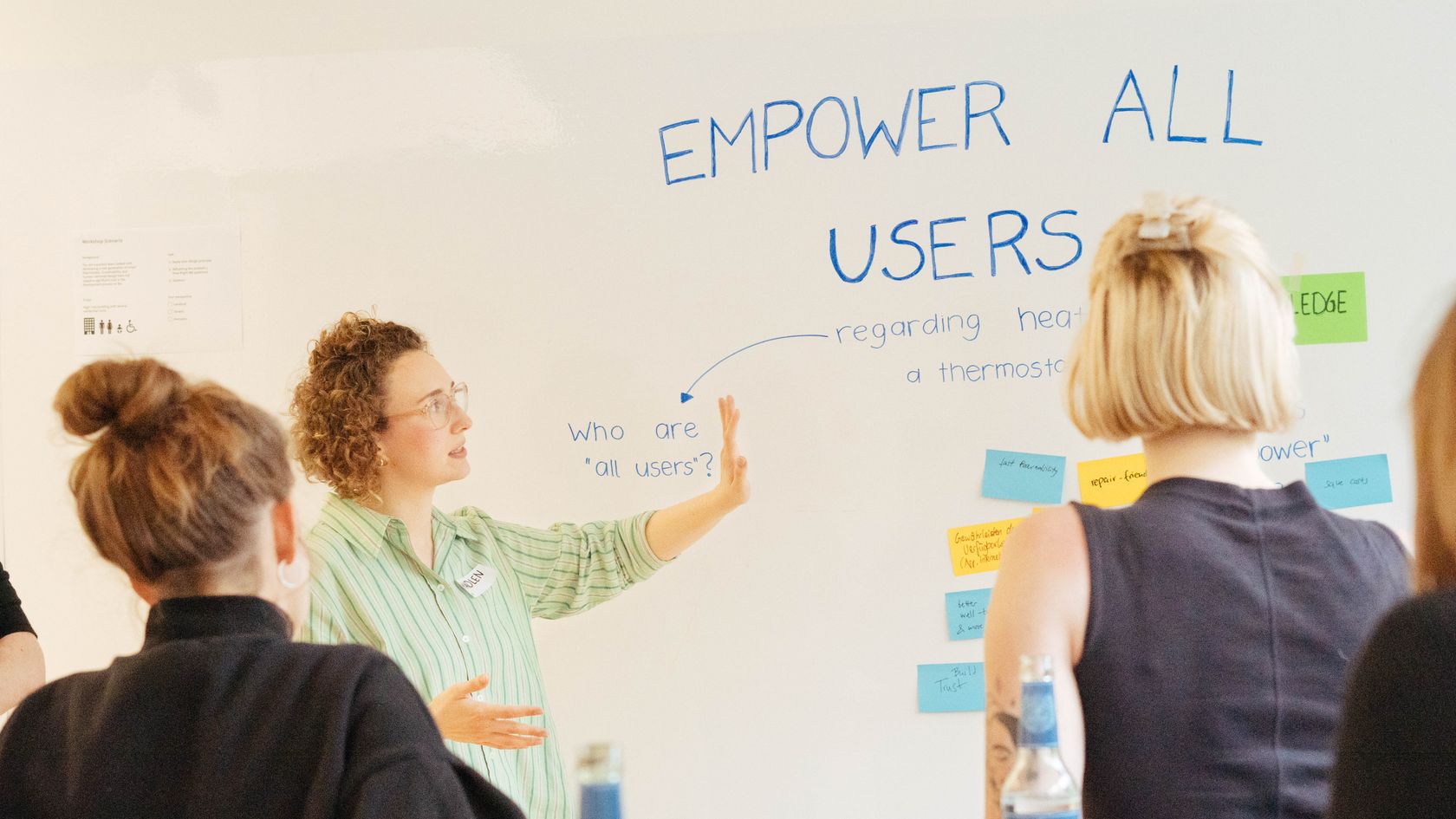
Proof-of-Concept insights with Ergosign
BriefMit KI Komplexität meistern
SIVAS.ERP is a comprehensive ERP system that covers almost all processes for mechanical and plant engineering companies. From sales to purchasing, production, and after-sales processes, the software offers a variety of functions and yet remains adaptable to different conditions and areas of application. But it is precisely this versatility that poses challenges: newcomers and inexperienced users often find it difficult to find their way around—from navigating through complex structures to using specific functions. The goal is to make the use of the software more intuitive, efficient, and user-friendly.
What could be more obvious than integrating a chatbot that could answer all questions about SIVAS.ERP? But we have set our sights on more! Chatbots are already a dime a dozen; we would like to go (at least) one step further and also look left and right! In addition to questions about the SIVAS software, the chatbot should also be able to answer questions about the entire operation. Who knows who to ask if you want to know how often vacation is filed on bridge days or what the lowest stock level of product X was within the last 12 months?
ApproachIdentifying potentials in an AI workshop
In a one-day AI Ideation Workshop with schrempp edv, we first analyzed all processes and user needs in depth. Thanks to the combination of our AI expertise and the insider knowledge of schrempp edv, we were able to achieve in a very short time:
Identify pain points in using software
Define potentials for AI implementation
Assess risks and feasibility
At the end of the workshop, we captured six promising AI project ideas. In addition to a chatbot that supports the use of the software, other ideas that came up were AI-generated playlists for explanatory videos and a chatbot for database queries in natural language, which helps pull information from the database without any knowledge of SQL (SQL = Structured Query Language). In this case study, we look at the chatbot as a concrete example of our methodology and process.
ApproachExamining feasibility and quality during the AI Exploration Sprint
SIVAS.ERP includes over 2,500 tables, 900 programs, and is used by over 5,000 users every day. This complexity makes it clear: a chatbot that knows all of the software's functions and can answer questions about it would have the potential to make everyday work significantly easier. However, it is not possible to judge at first glance whether the existing data is suitable for implementing such a chatbot.
That's why the Ideation Workshop was followed by the AI Exploration Sprint, in which we immediately tested the idea and implemented it in a proof of concept. Such a proof of concept enables a realistic evaluation of the idea based on real data. In this way, th
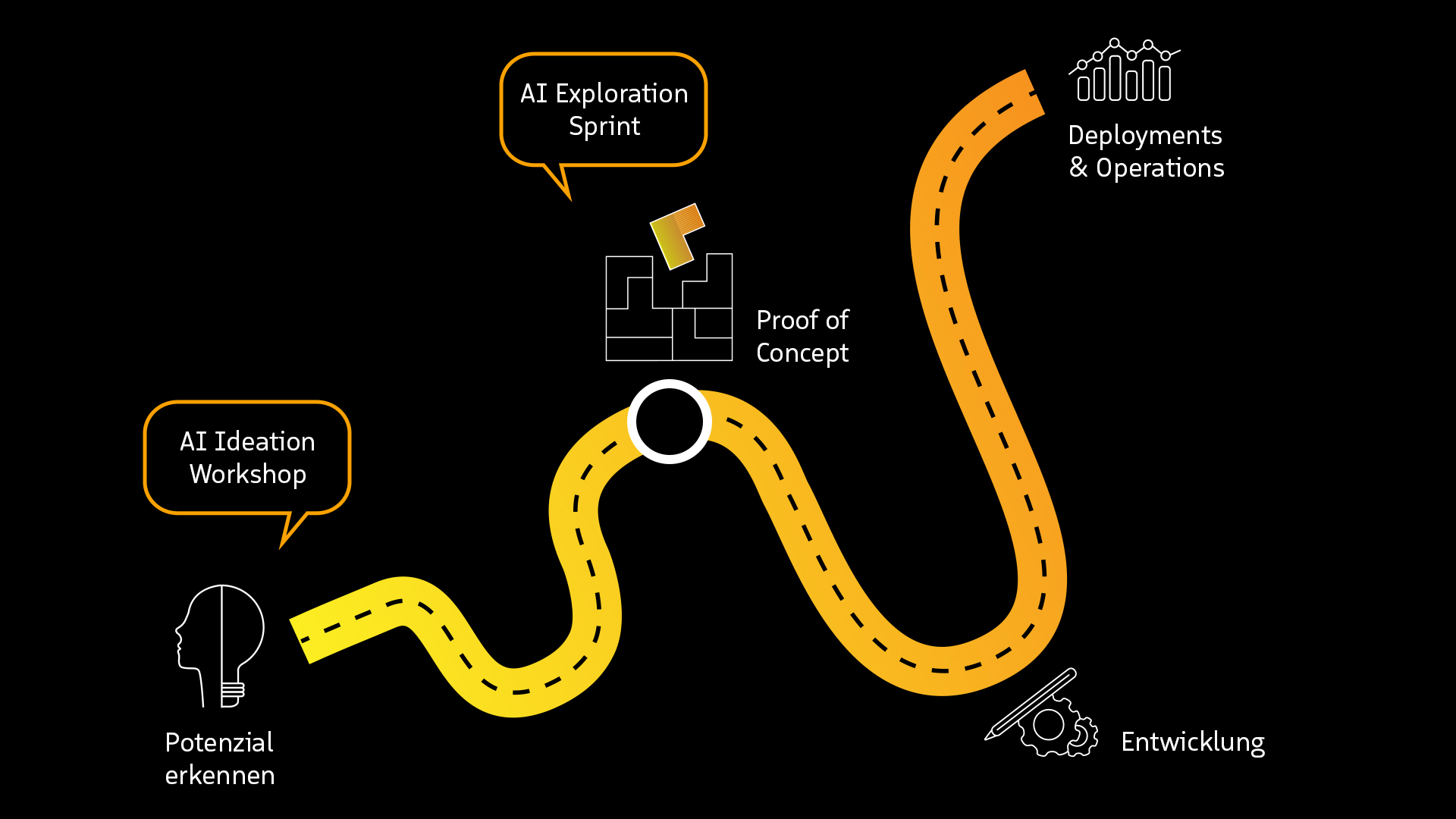
DigressionMethods
There are various methods of feeding a language model with internal company knowledge. Which method you choose depends on the application, the costs, and the required adaptability:
In-context learning: The model receives information directly through the prompt, which helps it answer the question or solve the task. The model is not changed, and you can quickly try out different things. However, the length of the input is limited.
Fine-tuning: This changes the model parameters through additional training on specific data, enabling permanent adjustments but being computationally intensive and expensive. In addition, the contents of the data cannot be changed later.
Retrieval-Augmented Generation (RAG): This approach combines a language model with a knowledge database from which relevant information is retrieved and made available to the model. Like in-context learning, the model can use the information provided to improve its answers without changing the model itself. The knowledge base can be updated at any time so that the latest information is always available.
OutcomeData analysis through SQL generation
A chatbot that makes internal company data accessible quickly and precisely would be an enormous relief for employees at all levels. It should answer questions like:
“In which month of the last two years did we have the highest sales of X? How has it developed since then?”
“How long does it take on average to reorder consumables X and Y?”
“How much overtime have we accumulated, and is that more or less than in previous years?”
We wanted to offer this support and developed a proof of concept for schrempp edv. Our system converts natural language into SQL queries, extracts the relevant information from the database, and prepares it in the desired form using a language model. This means users receive directly usable answers—whether as text, tables, or diagrams.
This represents a significant increase in efficiency, especially for teams without SQL knowledge, as important data is available more quickly and easily.
OutcomeA promising vision of the future
Within a few weeks, we developed several proofs of concept with extremely promising results in the AI Exploration Sprint for schrempp edv. We were able to impressively show that generative AI can offer real added value in the ERP area.
With our AI expertise and the right ideas, we help companies develop strategies for the sensible use of AI and put them into practice. This does not require a complete project order immediately—initial pilot projects are often enough to gain valuable insights.
About the clientschrempp edv
schrempp edv has been developing, selling, and supporting innovative, industry-optimized ERP systems for medium-sized mechanical and plant manufacturers since 1980. The flagship is the SIVAS.ERP software, for which we have implemented several AI ideas as a proof of concept. This project showed our customer how important it is to think about AI use from the beginning in the future and thus structure the data accordingly—we are already looking forward to the next steps!
Schrempp EDV was so excited about our concept and approach that they didn’t want to miss the opportunity to give a talk on the topic together with Sophia Antonin (Technology Manager AI & Data Science) at our DXC 2024.
Nehmen Sie an unseren kostenlosen Webinaren teil.
In unseren Ergosign Webinaren zum Thema KI und weiteren spannenden Themen präsentieren wir die neuesten Trends, bewährte Methoden und innovative Technologien.
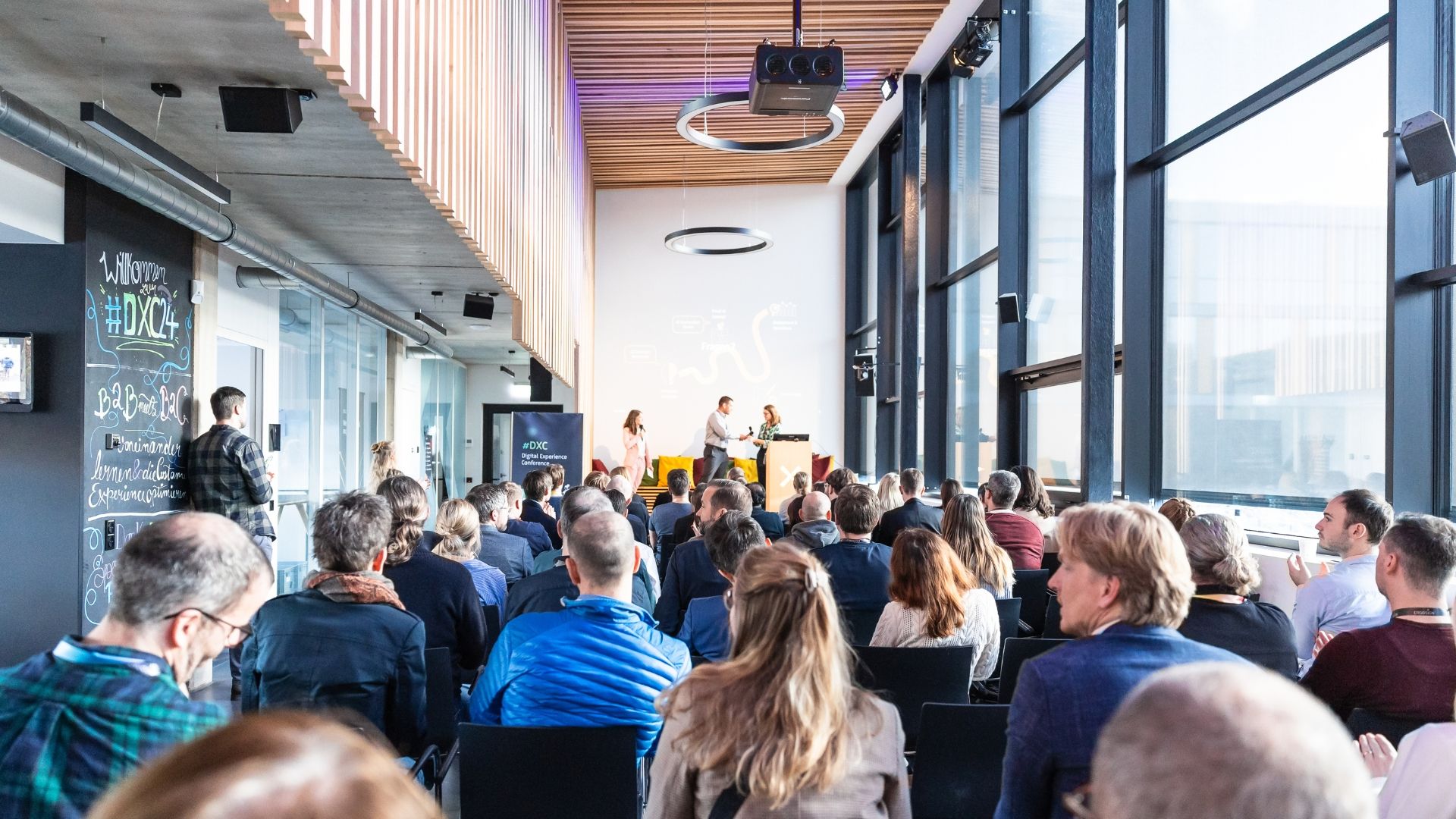
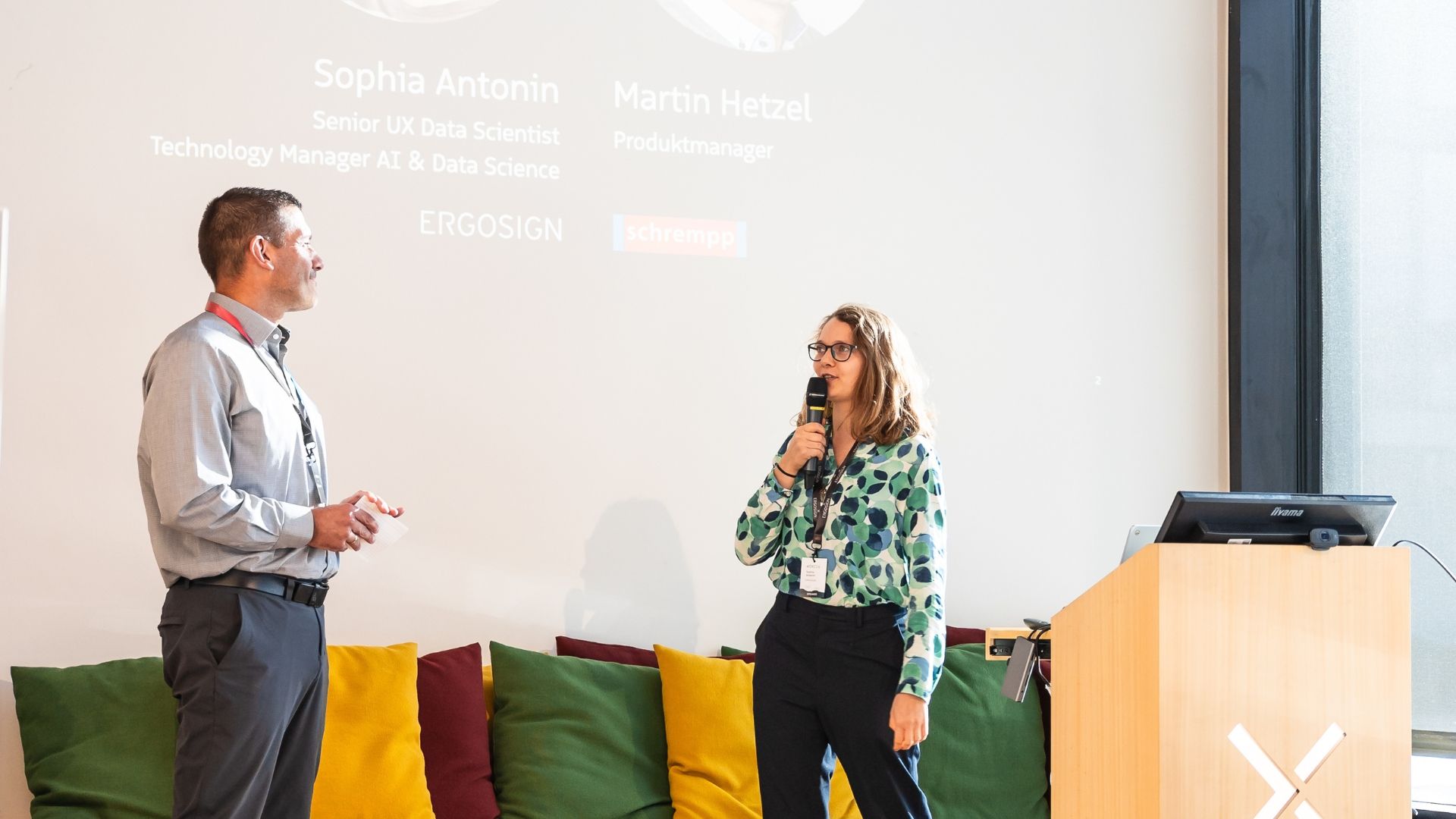
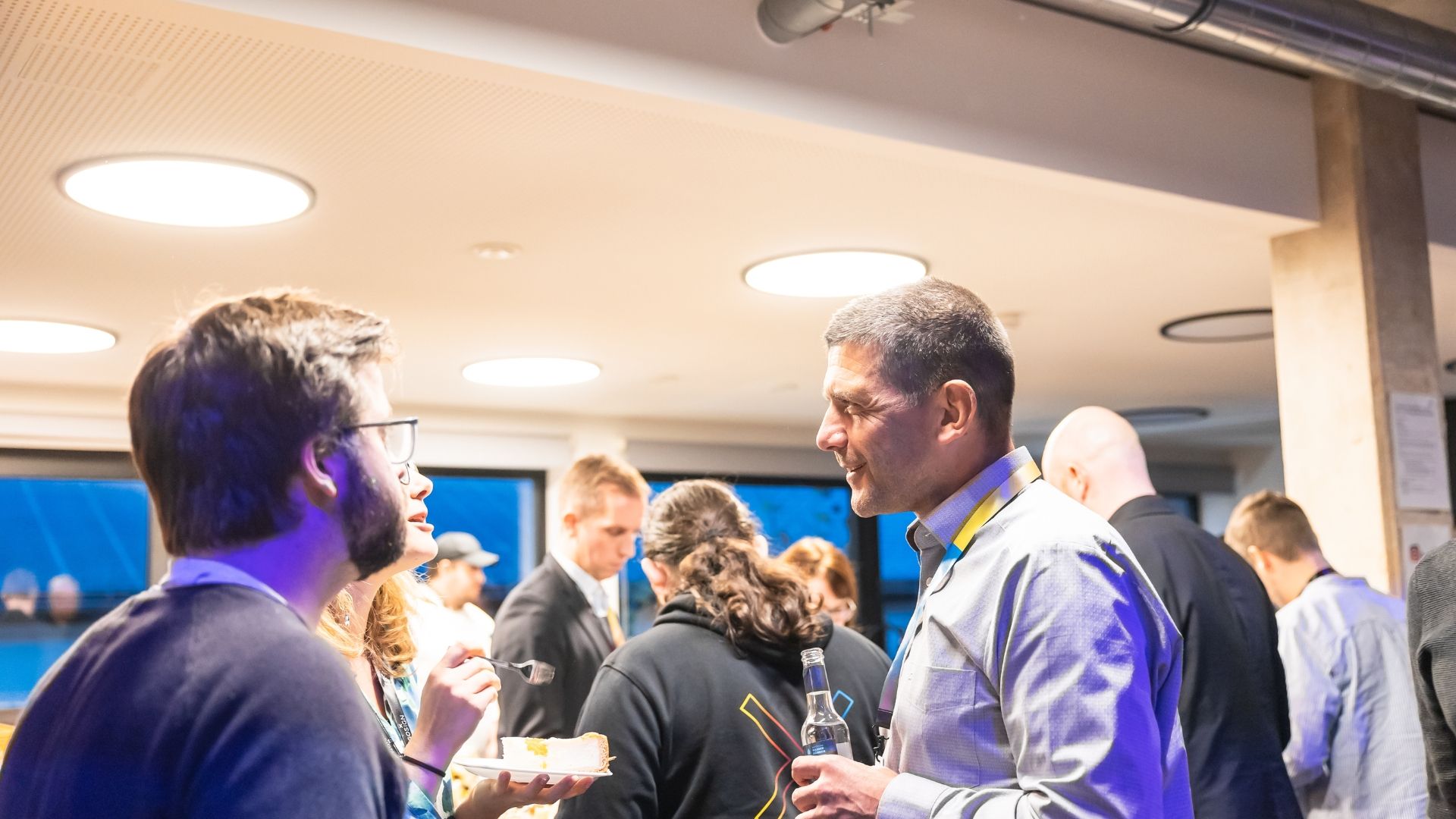
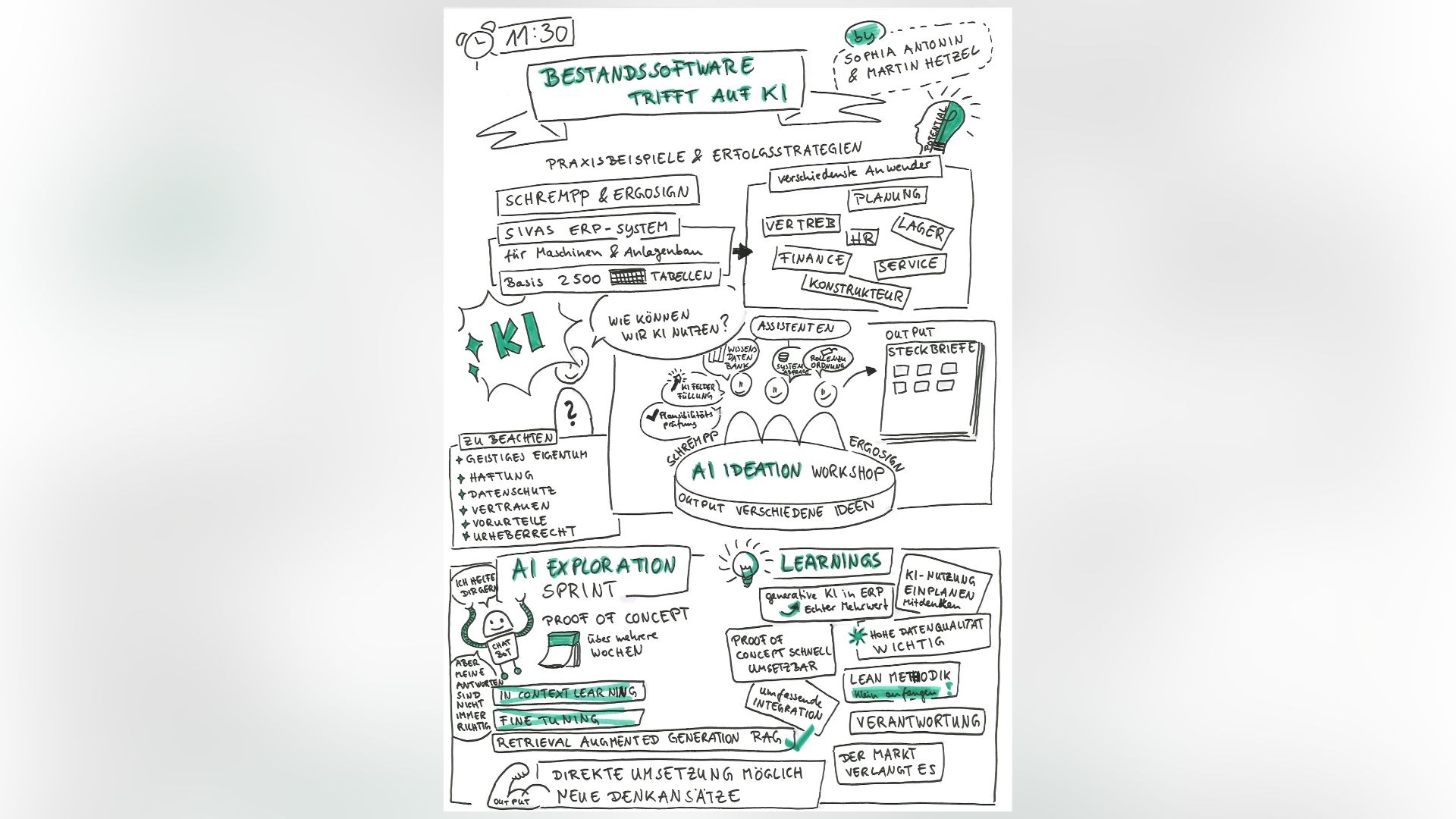